4D Datasets
4D Datasets
Epic Games has awarded Digital Air the first phase of a MegaGrant to produce high-resolution 4D human datasets for open-source research and standards development. When I was researching the needs of the research community I reached out to Dr. Michael Black with the idea. Here's what he said:
I agree that 4D datasets would be useful to the community.
Before developing a new dataset, however, one needs to really define what the big needs in the research community (are)? What is missing? What should the community focus on more? How could data advance this? What sort of evaluation/challenge would there be? What baseline algorithm(s) can be provided?
Dr. Michael Black
Max Planck Institute for Intelligent Systems
The final deck requesting the grant with our ideas about what is needed is linked below. We haven't started production yet so feel free to email us to request updates and let us know what features you'd like to see in the datasets.
The reason I reached out to Dr. Black about this was twofold: dating back to his pioneering PhD thesis on optical flow at Yale in 1992, Dr. Black is one of the most influential academics in the history of visual effects. Additionally, Dr. Black's research group at Max Planck Institute in Tübingen, Germany where he leads the Perceiving Systems Department, continues to do some of the most advanced applied research work in the world. For example, the rightmost mesh below, including the face, hands, and fingers, has been automatically estimated by Dr. Black's group -- with the help of machine learning from MPI's own datasets -- from the single 2D stock image on the left:
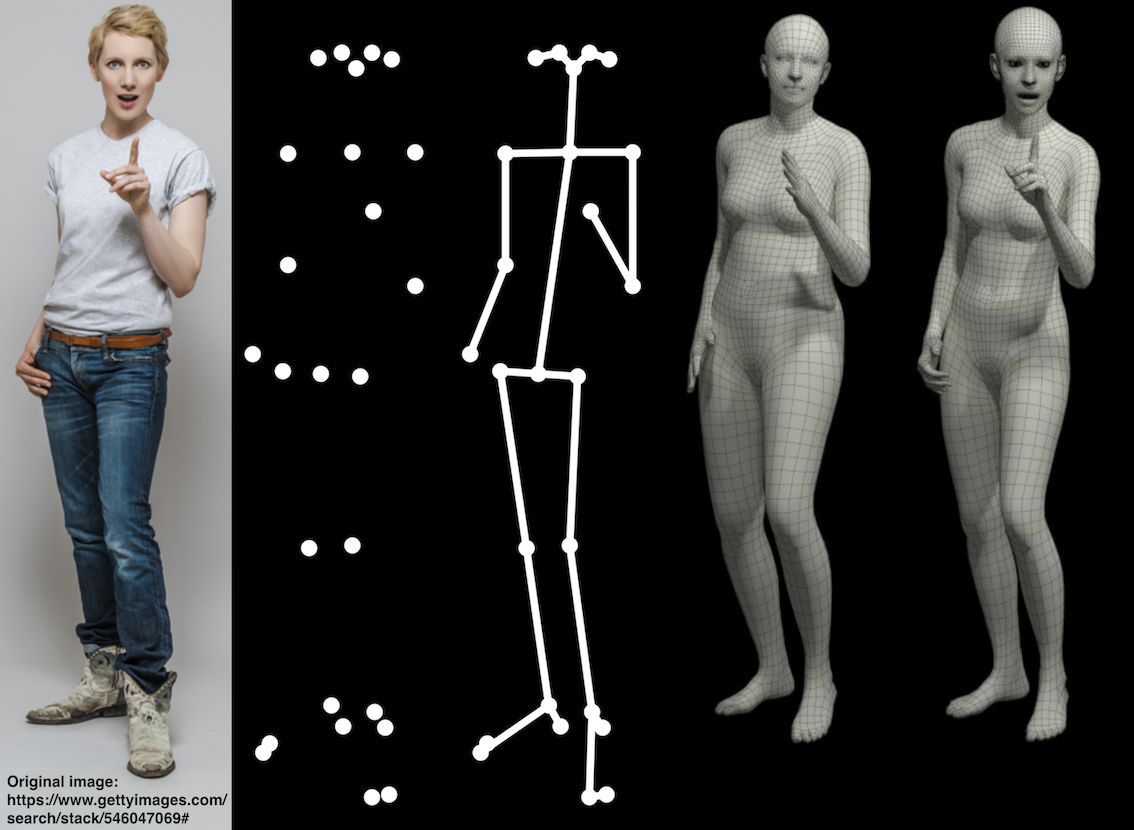
Amazing!
The research below is also applicable and impressive:
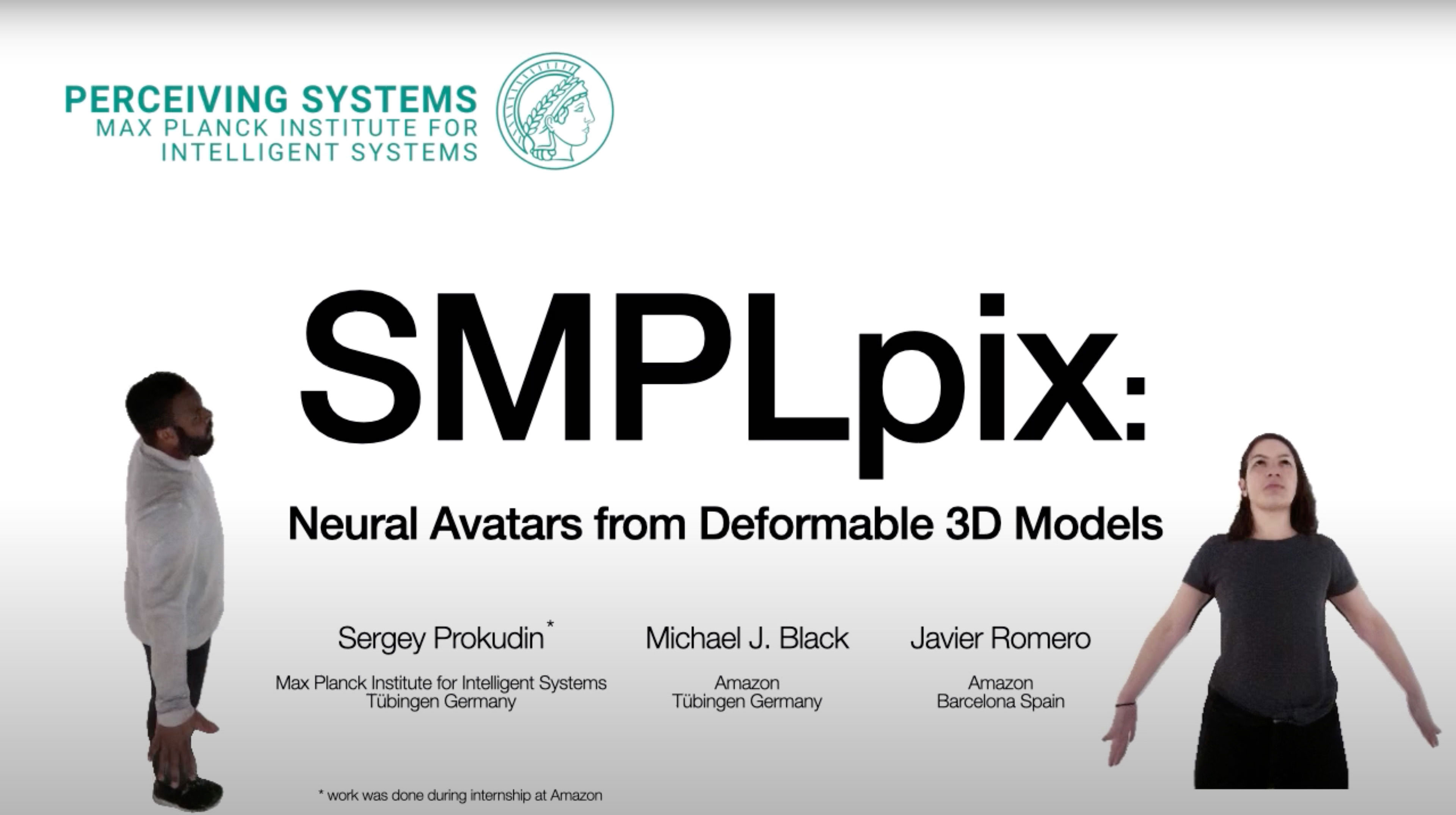
I've chosen to show the two examples above specifically because they are are from Dr. Black's research group at MPI. They are but two examples of ongoing research in computer graphics and perception around the world.
Unfortunately MPI's datasets are for non-commercial use only and are not suitable for photographic-quality 4D development as they lack usable photographic textures. Fully textured, dense, high-resolution photographic 4D datasets solved with markerless photogrammetry, as Digital Air has proposed, are needed. And to move the entire industry forward, those datasets need to be available to everyone in the community that has a constructive need for them, including commercial entities.
Processes for producing playable 4D assets derived from live-action that can be automatically retopologized with temporally consistent meshes, automatically adjusted to fit the original 4D mesh sequences at every frame, and then reanimated by graphics engines will depend upon robust datasets for development, testing, and refinement. Comparative results that demonstrate methods and improvements based on freely available input data will be needed, as well as open standards to link 4D processes from acquisition to playback across commercial workflows. When it all comes together, ideally in the form of open standards for playable 4D assets at any resolution in which walk cycles can be adjusted into run cycles and other basic movements can similarly be adjusted and enhanced, visual effects workflows and animation engines are immediately going to break the realism of the original data as they push it beyond the natural limitations of the original performances, providing new problems for computer vision and machine learning scientists to solve.
If your work and or interests relate to 4D data and machine learning and you would like more information feel free to read through the deck below and email us with your comments and to sign up for progress reports.
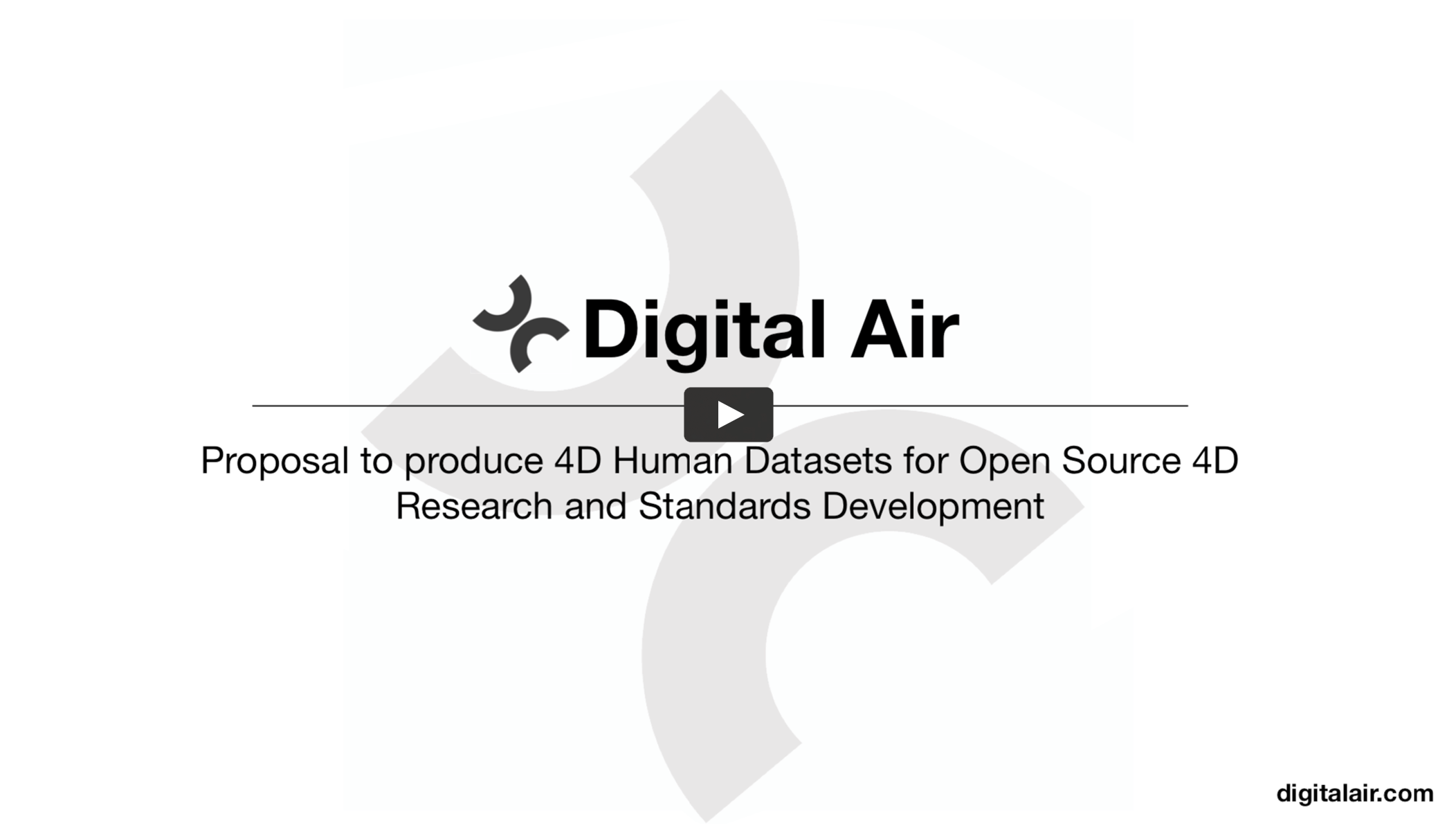
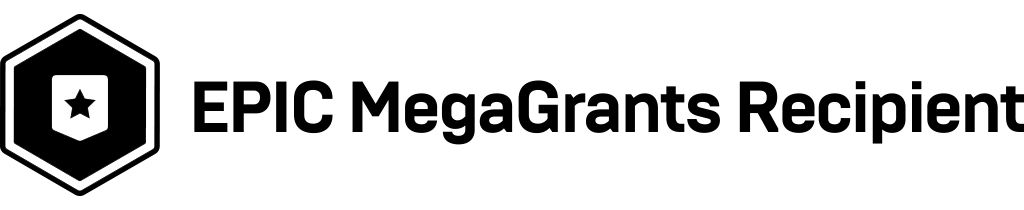
Update May 24, 2021
The draft dataset specifications below are now open for comments. Please feel free to email us with your feedback.
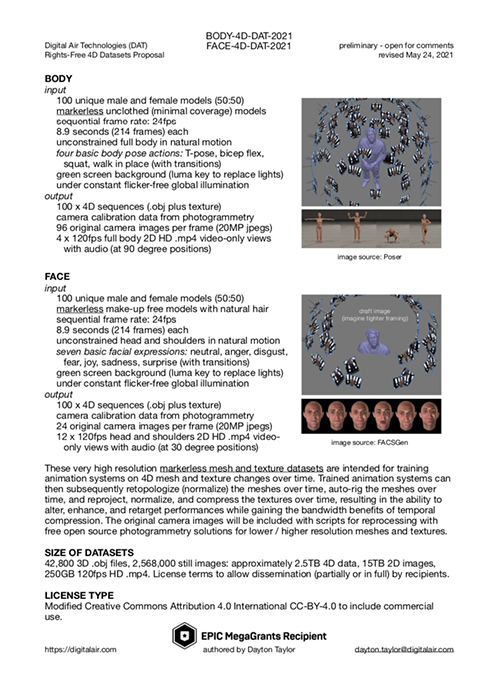